Share this
Domain Generation Algorithms: The Cybersecurity Arms Race at the DNS Layer
by Kory Underdown on Apr 30, 2025 7:15:00 AM
Imagine this: Your firewall is blocking thousands of threats every single day. Your endpoint protection is flagging suspicious files left and right. On paper, your defenses look bulletproof. But somewhere, quietly and invisibly, malware is slipping through the cracks—using a tactic so clever it’s practically hiding in plain sight.
These algorithms churn out thousands—sometimes tens of thousands—of random domain names every day, giving attackers an endless supply of digital “safe houses” to communicate with their malware. By the time you’ve blocked one domain, the algorithm has already moved on to the next.
The result? It takes the average organization 258 days to detect and contain a breach. That’s about 8 months of stolen data, compromised systems, and mounting costs—all because DGAs exploit one of the Internet’s most fundamental tools: DNS, the system that translates website domain names into IP addresses.
This isn’t just a technical problem; it’s a strategic one. DGAs are like an opponent in chess who changes the rules mid-game, forcing you to play catch-up while they stay three moves ahead. To win this battle, you need more than reactive defenses—you need to rethink how you protect your network at its core.
What Makes DGAs the Ultimate Cybersecurity Chess Move
If malware were a chess player, Domain Generation Algorithms (DGAs) would be its grandmaster strategy. They’re not brute force attackers—they’re clever, adaptive, and always thinking three moves ahead.
Here’s how they work: Random domain names generated by DGAs act as temporary safe houses for malware to communicate with its command-and-control (C2) servers. Once a domain is blocked, the algorithm simply spins up a new one, leaving defenders scrambling to keep up.
Why does this work so well? Because DGAs exploit a fundamental vulnerability in the Internet’s architecture: DNS (Domain Name System). DNS is like the Internet’s phonebook—it translates human-friendly website names (like “example.com”) into machine-readable IP addresses. But here’s the catch: DNS was built on trust, not interrogation. It assumes that every query is legitimate, which makes it an easy target for attackers who know how to game the system.
And it’s not just about volume—it’s about evolution. Early DGAs relied on predictable patterns like timestamps to generate domains, making them relatively easy to spot. But modern variants have leveled up, using machine learning and environmental data (like network traffic patterns or even weather updates) to create domains that are harder to detect and block.
To beat DGAs, defenders need more than reactive measures—they need predictive solutions that can outthink the algorithms themselves.
Why Traditional Defenses Fail Against DGAs
The truth is most cybersecurity defenses weren’t built to handle the chaos DGAs create. These algorithms are like digital escape artists, exploiting every blind spot in your security stack. The problem isn’t that defenders aren’t trying—it’s that traditional tools are playing by rules that DGAs broke years ago.
Traditional firewalls focus on blocking unauthorized access through known ports and protocols, but they often overlook DNS traffic—a critical vulnerability attackers exploit. Malware uses DNS to create hundreds of randomized domains daily to bypass blocklists. As highlighted in The DNS-Based Threats Your Firewall Ignores, these gaps leave organizations exposed to stealthy attacks that firewalls simply weren’t designed to catch.
The 3 Fatal Blind Spots
- Static Blocklists Are Outdated: Imagine trying to stop a flood with a bucket. That’s what static blocklists do against DGAs. These lists rely on pre-identified malicious domains, but DGAs generate thousands of new ones every day—most lasting less than 24 hours. By the time you’ve added one domain to the list, the algorithm has already moved on to the next 10,000.
- Signature-Based Tools Miss the Mark: Traditional antivirus and intrusion detection systems rely on signatures—patterns of known malware activity—to flag threats. But DGAs don’t play by predictable patterns.
- Endpoint Protection Falls Short: Even endpoint detection and response (EDR) tools struggle with DGA traffic because it mimics legitimate DNS activity.
Detecting DGAs: From Needle-in-Haystack to Predictive DNS Defense
Detecting DGAs is a bit like spotting counterfeit bills in a stack of cash—it’s doable, but only if you know what to look for. Attackers design these algorithms to blend into legitimate DNS activity, making them hard to identify with traditional methods. But defenders are fighting back with smarter, layered approaches that combine pattern recognition, behavioral analysis, and machine learning.
Step 1: DNS-Layer Inspection
The first step in detecting DGAs is analyzing DNS traffic itself. This involves looking for patterns that suggest randomness or unusual activity. For example, domains generated by DGAs often exhibit high entropy (a measure of randomness) because they’re designed to evade detection. Techniques like N-Gram analysis can help calculate a randomness score for domain names, flagging those that deviate from typical human-generated structures.
Machine learning models also play a key role here. By training algorithms to recognize suspicious domains based on historical data, defenders can classify domains as potentially DGA-generated. Deep learning approaches, such as autoencoders and classification models, are particularly effective at profiling DNS traffic and identifying anomalies in real-time.
Step 2: Network Behavior Red Flags
Sometimes it’s not the domain itself but the behavior surrounding it that reveals malicious activity. For instance, here are two issues to be on the lookout for:
Failed query patterns: DGA-generated domains often fail to resolve because they’re designed to test multiple options until one connects to a command-and-control (C2) server. Frequent failed DNS queries can be a strong indicator of DGA activity.
Unusual traffic spikes: Malware using DGAs often generates bursts of DNS queries during off-peak hours when network activity is minimal, making these anomalies easier to spot.
Be Aware of the "Alert Paradox"
Even with advanced detection methods, alerts can become overwhelming—especially when they’re ignored during off-hours. This is known as the “3:30 AM problem,” where valid alerts go unnoticed because security teams are stretched thin or fatigued by false positives. Contextual scoring systems help solve this by prioritizing alerts based on urgency (e.g., 10+ nonsense domains queried in 5 minutes triggers a P1 alert).
The Positive Ripple Effect of DNS Inspection
The benefits of DNS-layer defenses go far beyond just catching threats in real time. Organizations using AI-powered DNS filtering neutralize threats faster, reduce false positives, and achieve deeper visibility into attack infrastructures.
Because DGAs often rely on domain randomness and burst traffic, DNS inspection provides a high-signal detection point that other layers miss. By analyzing anomalies and scoring domains in real-time, security teams can surface threats earlier—sometimes before malware has a chance to fully deploy.
And when DNS-layer visibility is paired with behavioral context—such as failed resolution patterns, traffic spikes, or sandbox triggers—the result is faster threat attribution, better alert prioritization, and tighter coordination across the security stack.
Proactive DNS inspection turns what used to be a reactive clean-up operation into a preemptive strike.
DNSFilter is at the forefront of this evolution, delivering intelligent protections that stop AI-driven threats—like DGAs, typosquatting, phishing, and other evasive malware—before they ever reach your endpoints.
Building a DGA-Resistant Architecture
Stopping DGAs isn’t about patching holes—it’s about building a fortress. Attackers are constantly evolving their tactics, so your defenses need to be proactive, adaptable, and layered. A DGA-resistant architecture doesn’t just block malicious domains; it anticipates them, disrupts their operations, and minimizes the fallout when something slips through.
The 4-Pillar Framework
- Preemptive DNS Filtering: Think of this as your first line of defense. Advanced DNS filtering tools use machine learning to score domains in real-time, flagging those with high probabilities of being DGA-generated. For example, if a newly queried domain looks random or matches known DGA patterns, it can be blocked before it’s ever resolved.
- Context-Aware Sandboxing: Sandboxing isolates suspicious activity in a controlled environment to observe its behavior without risking your network. For instance, if a PDF download triggers hundreds of DNS queries in seconds, sandboxing can determine whether the activity is benign or part of a DGA-driven attack.
- Threat Intelligence Fusion: Attackers thrive on unpredictability, but threat intelligence turns randomness into patterns. By correlating data from global threat feeds, historical attack trends, and machine learning models, defenders can identify emerging DGA tactics and preemptively block them before they spread.
- Automated Takedown Workflows: Speed matters in cybersecurity. Automated workflows can instantly block malicious domains at the registrar level or update DNS filtering rules across your network as soon as new threats are identified—no manual intervention required.
By 2025, Zero Trust will no longer be optional—it will be the dominant security model, replacing outdated perimeter-based approaches. As noted in 2025 Cybersecurity Predictions: It’s Not Just AI, Protective DNS filtering aligns perfectly with Zero Trust principles by proactively blocking malicious domains before they can infiltrate your network.
Stop DGAs Before They Start: Smarter DNS Security with AI
At DNSFilter, we’re building intelligent, adaptive defenses that detect and block DGA-generated domains in real time and before they’re used in attacks. By applying advanced machine learning at the DNS layer, we identify threats that haven’t been seen before, giving organizations a critical advantage against fast-evolving malware.
Our approach shortens response times, reduces false positives, and neutralizes threats before they can escalate, keeping networks safer without adding complexity.
AI-powered DNS security isn’t just the future—it’s how you stay ahead today. Start your free trial of DNSFilter and see how proactive DNS protection makes all the difference.
Share this
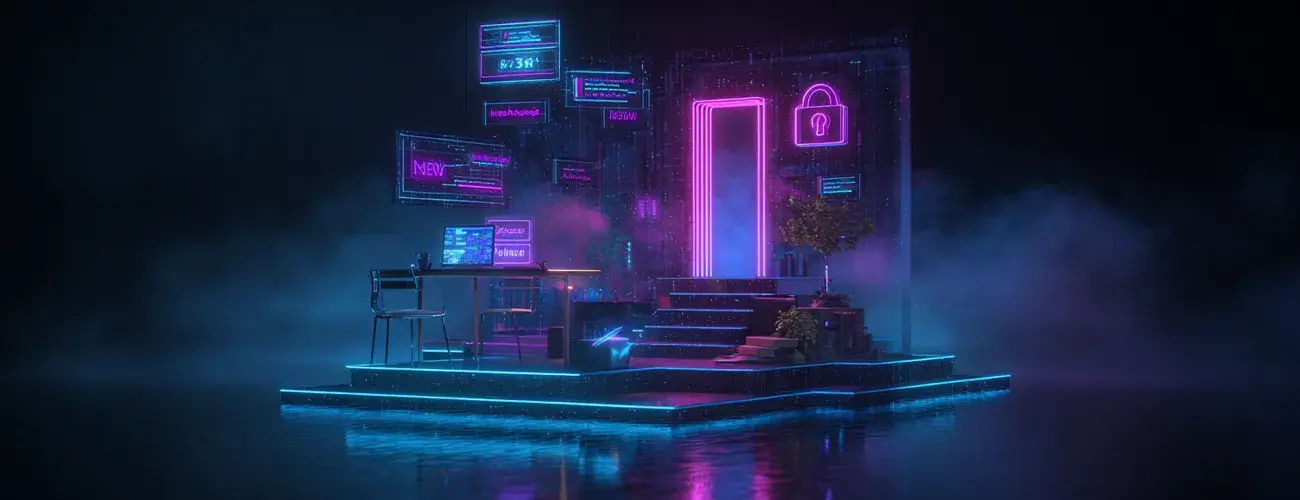
Our analysis of Tycoon 2FA infrastructure has revealed significant operational changes, including the platform's coordinated expansion surge in Spanish (.es) domains starting April 7, 2025, and evidence suggesting highly targeted subdomain usage patterns. This blog shares our findings from analyzing 11,343 unique FQDNs (fully qualified domain names) and provides 65 root domain indicators of compromise (IOCs) to help network defenders implement mo...
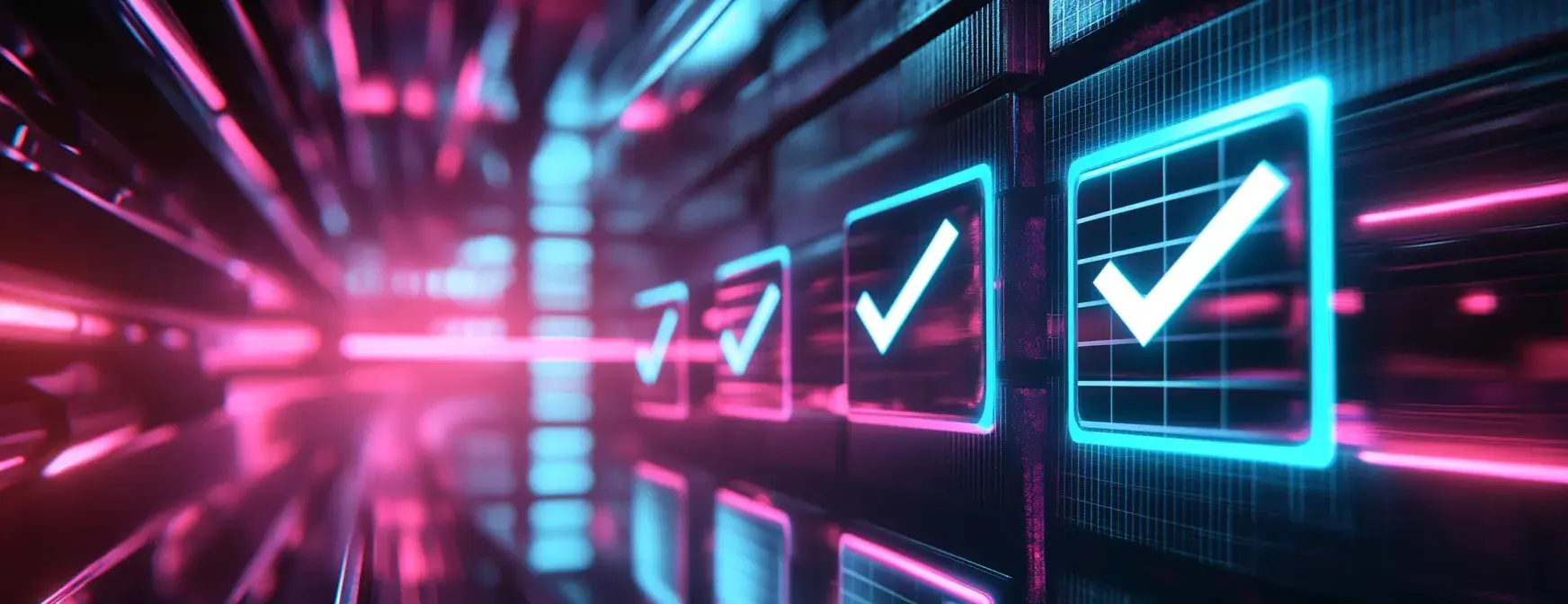
Staying Ahead with Smarter Web Filtering
Across every industry and network environment, content filtering isn’t just a matter of productivity, it’s a front line of defense. From malware and phishing to compliance risks and productivity drains, the threats are real, and the stakes are high.
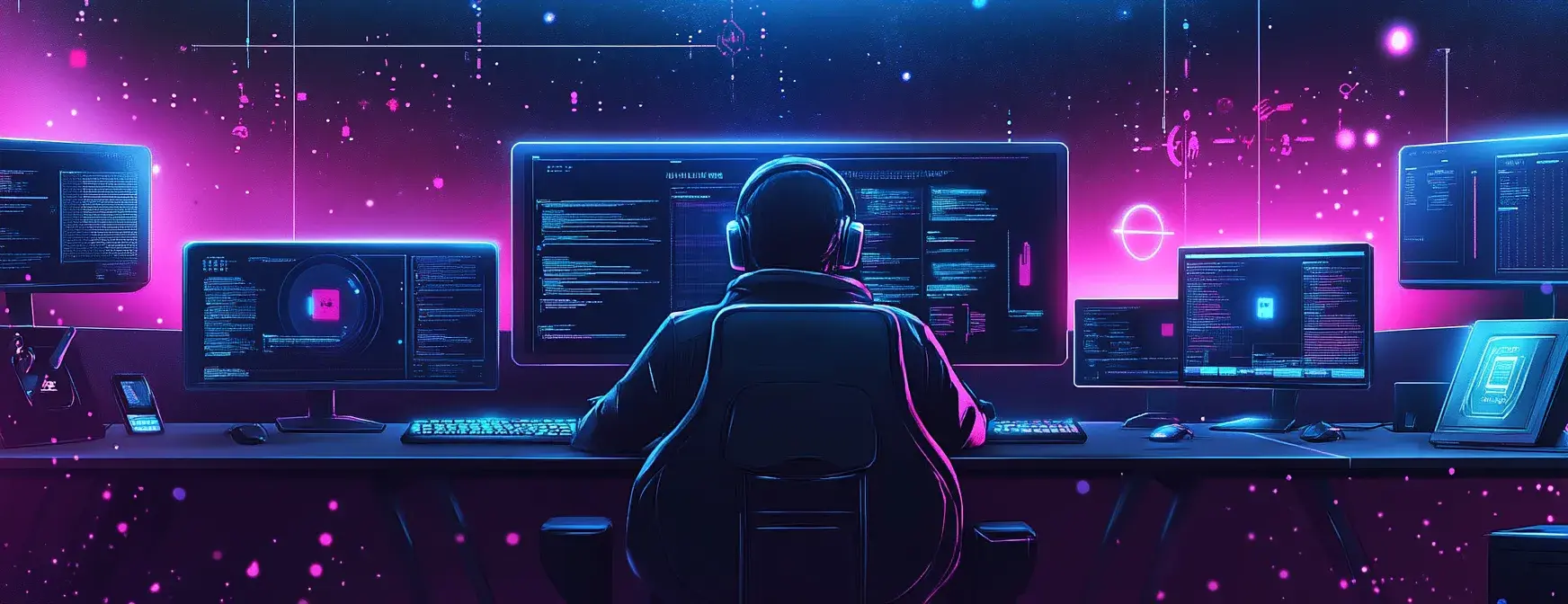
DNS filtering is a foundational layer of defense and helps to fortify the strongest security stacks. Most organizations use DNSFilter to block the obvious: malware, phishing, and adult content. That’s a great start, but many are missing out on the broader potential of DNS policies.