Share this
Analyzing a Third-Party Threat Feed Portfolio
by Henri Laakso on Jun 10, 2022 12:00:00 AM
Like most cybersecurity providers, DNSFilter supplements its in-house data with third-party threat intelligence. This comes from a collection of more than 60 different paid and open sources, amounting to millions of reports on domains.
It is important for us, like any organization, to regularly analyze our portfolio of threat intelligence. This article covers a range of techniques to gain insight into our collection of third-party threat intelligence.
Comparing threat feeds by size
The DNSFilter collection of third-party threat intelligence covers a large span of feeds, by size, as seen in Fig. 1 (below). This is to be expected as feeds are generated via different techniques, cover different threat types, and have different levels of focus.
How does DNSFilter compare domains?
When comparing domain name threat data, it is important to choose which label-level this is done at.
For example, say we have two Fully Qualified Domain Names (FQDNs) www.malicious.freesite.host
and foo.malicious.freesite.host
. Should these be considered overlapping pieces of threat intelligence?
It can be useful to look at the highest blockable label, which in this case would be malicious
as the second-level domain freesite.host
is a hosting service.
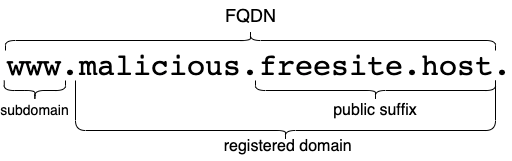
For this reason, for the rest of the article 'domain' is used to refer to the domain name down to the highest blockable label. Otherwise FQDN is used.
In this context, it can also be of interest to look at the amount of reported domains and FQDNs which are unique to each feed. Let’s use Feed Q as an example. Almost all the FQDNs in Feed Q are unique but the domains are mostly overlapping with other feeds. It could be further analyzed if this feed is bringing value and if these subdomains are blockable at a higher level.
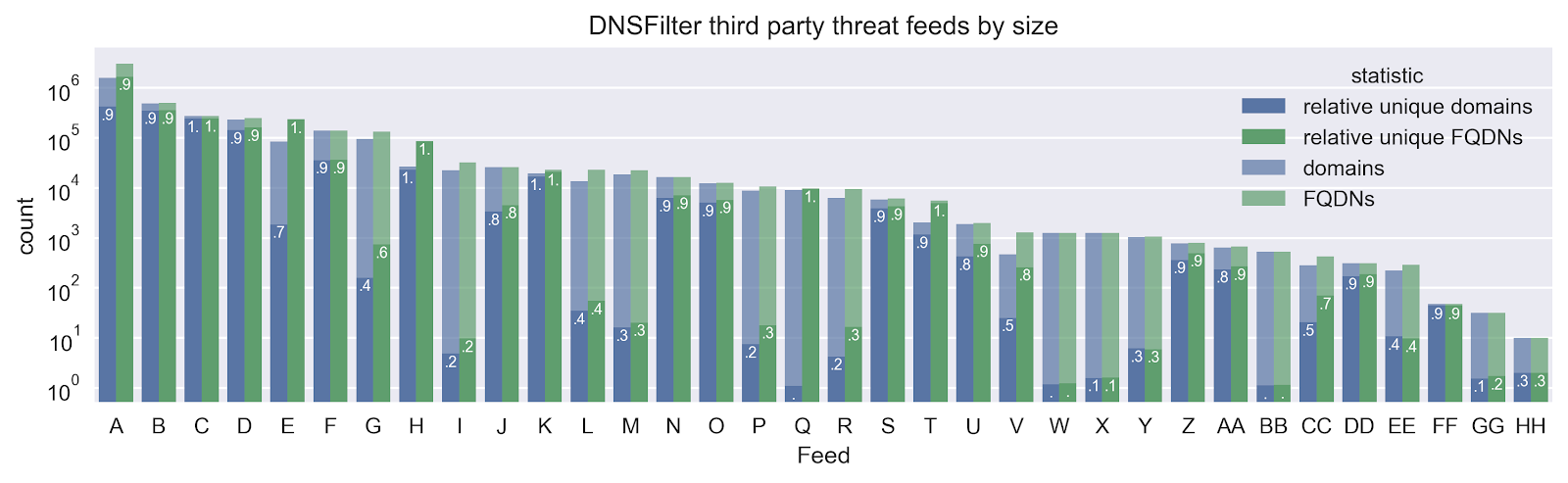
Figure 1. Comparison of threat feed by size by count of domains and FQDNs. Note the y-axis is logarithmic. The relative unique ratio shows the ratio of each feed that is unique to the feed. This is not represented logarithmically, but as a ratio of the total.
How fresh is your threat intelligence?
It is important to keep fresh intelligence, as stale data can be irrelevant or, worse, inaccurate. Many threat intelligence sources do not age out their data so this is left to the customer. As time goes by, the chances of a false positive increase.
From Figure 2, it is clear that the vast majority of DNSFilter's third-party threat data is fresh. The vast majority of domains were added in the last few months, with a small number of domains older than a few months. Spikes on specific days can correspond to new feeds, which are continually added by our team.
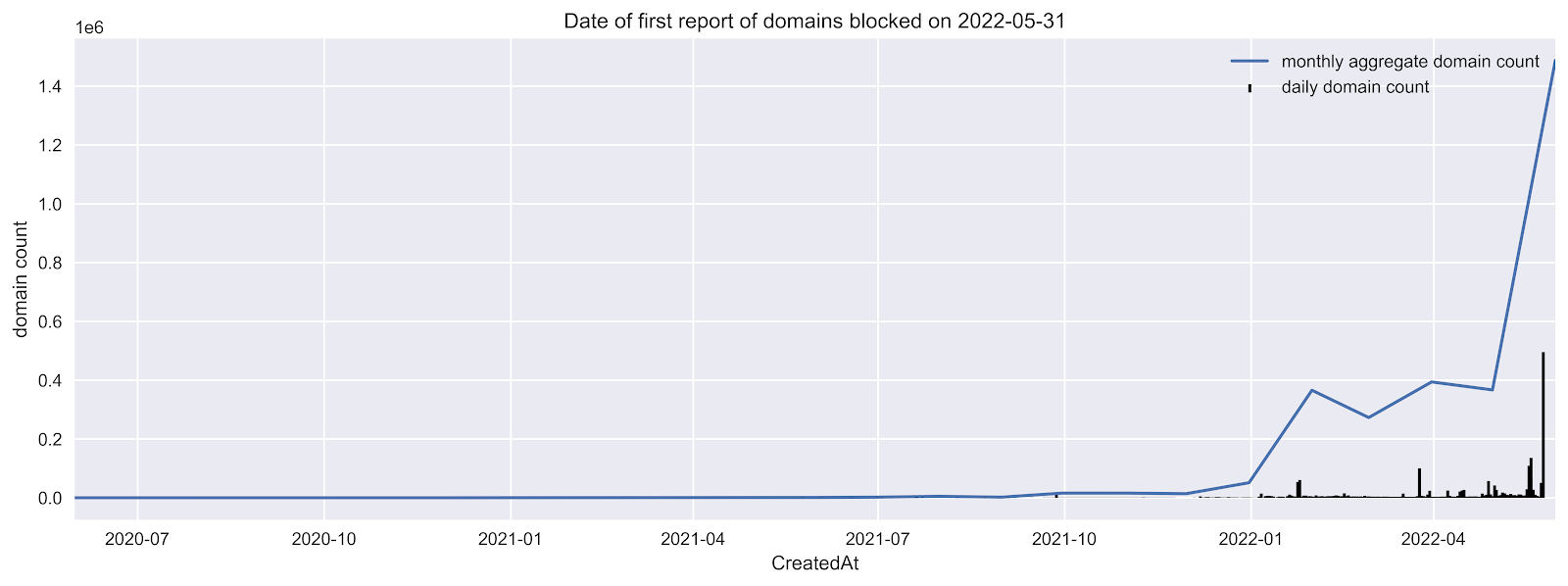
Figure 2. Month of first report for domains currently blocked by DNSFilter due to third-party reports.
We can break this down by threat category. As expected malware and botnet C2 domains are transient and age out quickly. Deceptive domains, which include phishing and scam domains, age out over a period of several months as campaigns are rolled out.
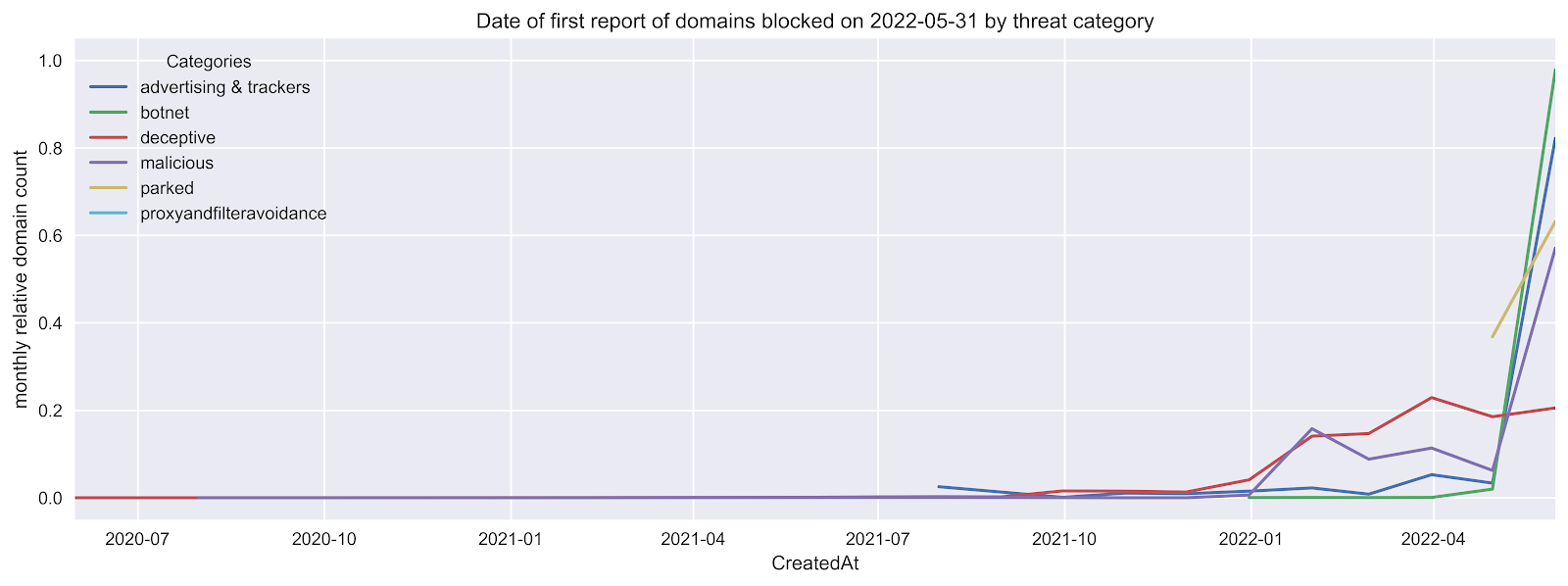
Figure 3. Month of first report for domains currently blocked by DNSFilter due to third-party reports. The counts are normalized for each threat type.
Do your threat feeds overlap?
A closer look at how feeds overlap shows a very sparse set of data, with a few exceptions. Figure 4 shows very little consistent overlap between feeds. Even for the largest feed, Feed A, the majority of the overlaps are below 20% of the smaller feed.
In some very specific cases, such as Feed W & Feed X, the overlap is significant. This is understandable, as they aim to cover the same specific threat actors. This and other significant overlaps could also represent data sharing agreements.
The figure shows the reasoning for using a multitude of feeds—the threat intelligence space is very sparse. This result is consistent with many other similar studies, which indicate that a single source of ground truth does not exist.
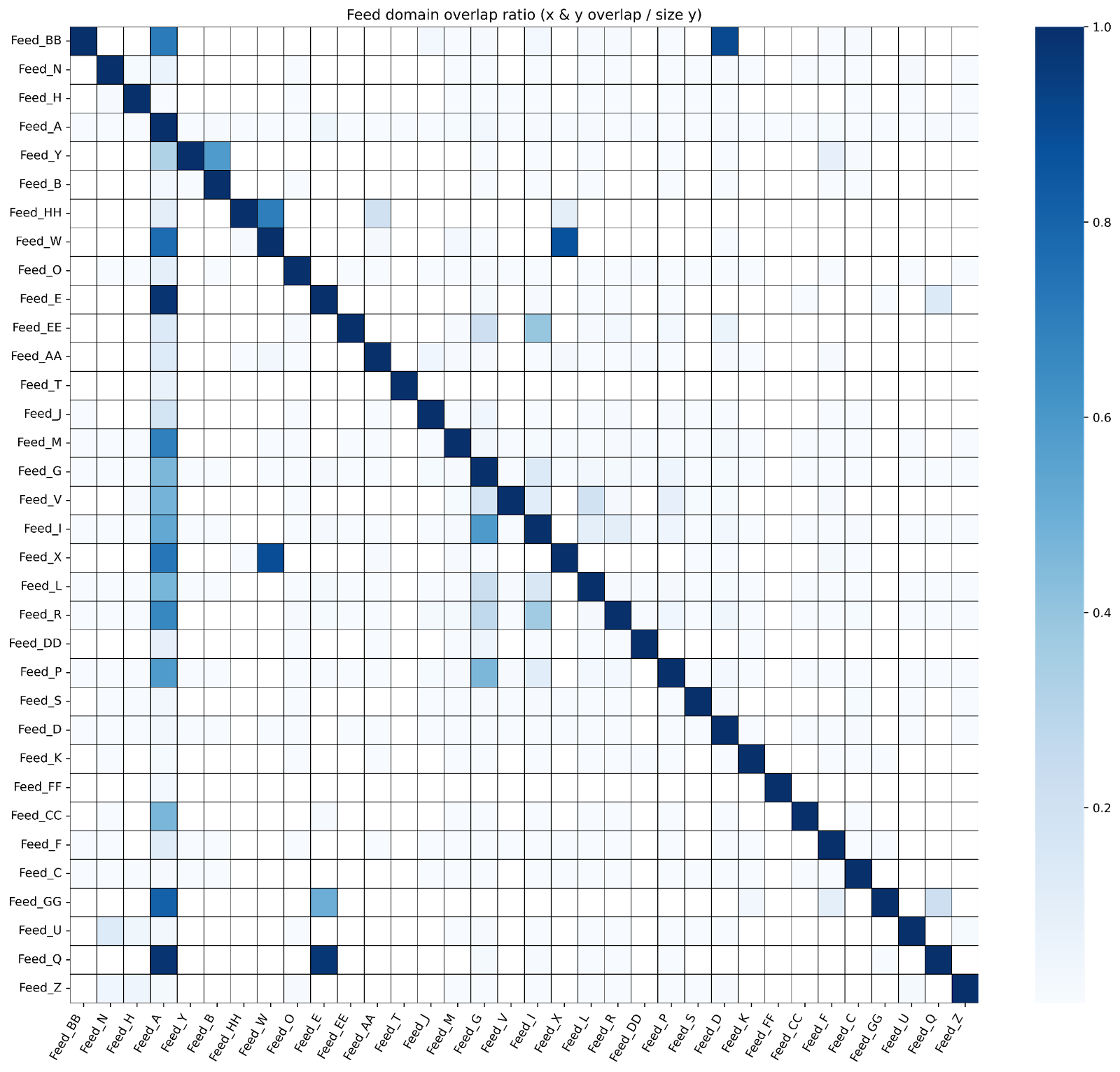
Figure 4. Overlap between domains in feeds. The color of each cell represents the ratio of overlapping domains to the size of the feed on the y-axis. For example, the overlap of feeds Y and B is a much larger ratio of the total size of feed Y as shown by a darker color.
Delays in reporting between feeds
A static overlap analysis does not tell the whole truth, as it does not take timing into account. As timeliness is an important factor in actionable intelligence, this should be measured.
Figure 5 shows that most overlaps between feeds occur within zero or one days of the initial report. Roughly 50% of overlapping reports occur in the first three weeks. For cases longer than this, it is likely domains become non-malicious and then are caught again later. Large spikes in some cases indicate specific data sharing agreements between feed providers.
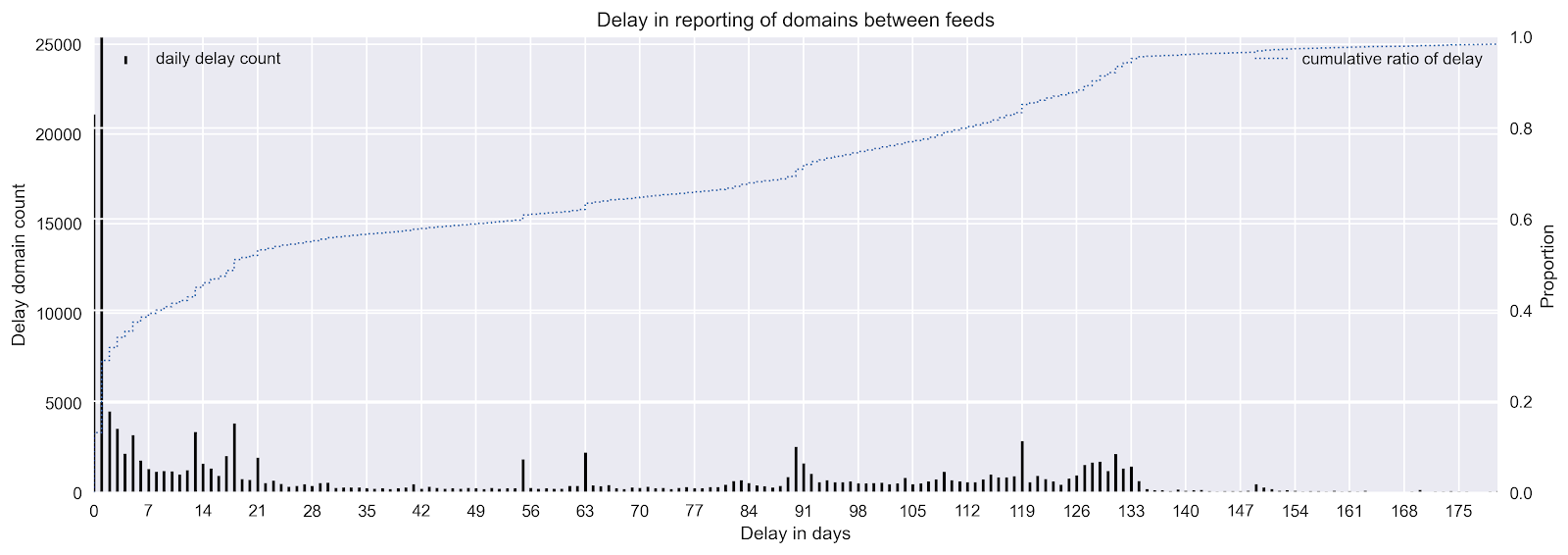
Figure 5. Delay in reporting time between feeds for the same domain. The count of overlapping domains with x days of delay is shown by the black stems. The blue line shows a cumulative ratio of the count of overlapping domains with x days delay.
We can further break this down by individual feeds to see which ones are lagging (positive delay) and which leading (negative delay). This is shown for larger feeds in Figure 6.
The transfer of data between feeds W and X can be observed here again, occurring with a roughly 20 day delay in most cases.
The largest feed, Feed A can be seen leading in most overlap cases, showing the value of the feed.
Other feeds like feed J, have no clear relationship with other individual feeds, and the delay pattern resembles a poisson distribution, generally a result of a random process, although with a small skew.
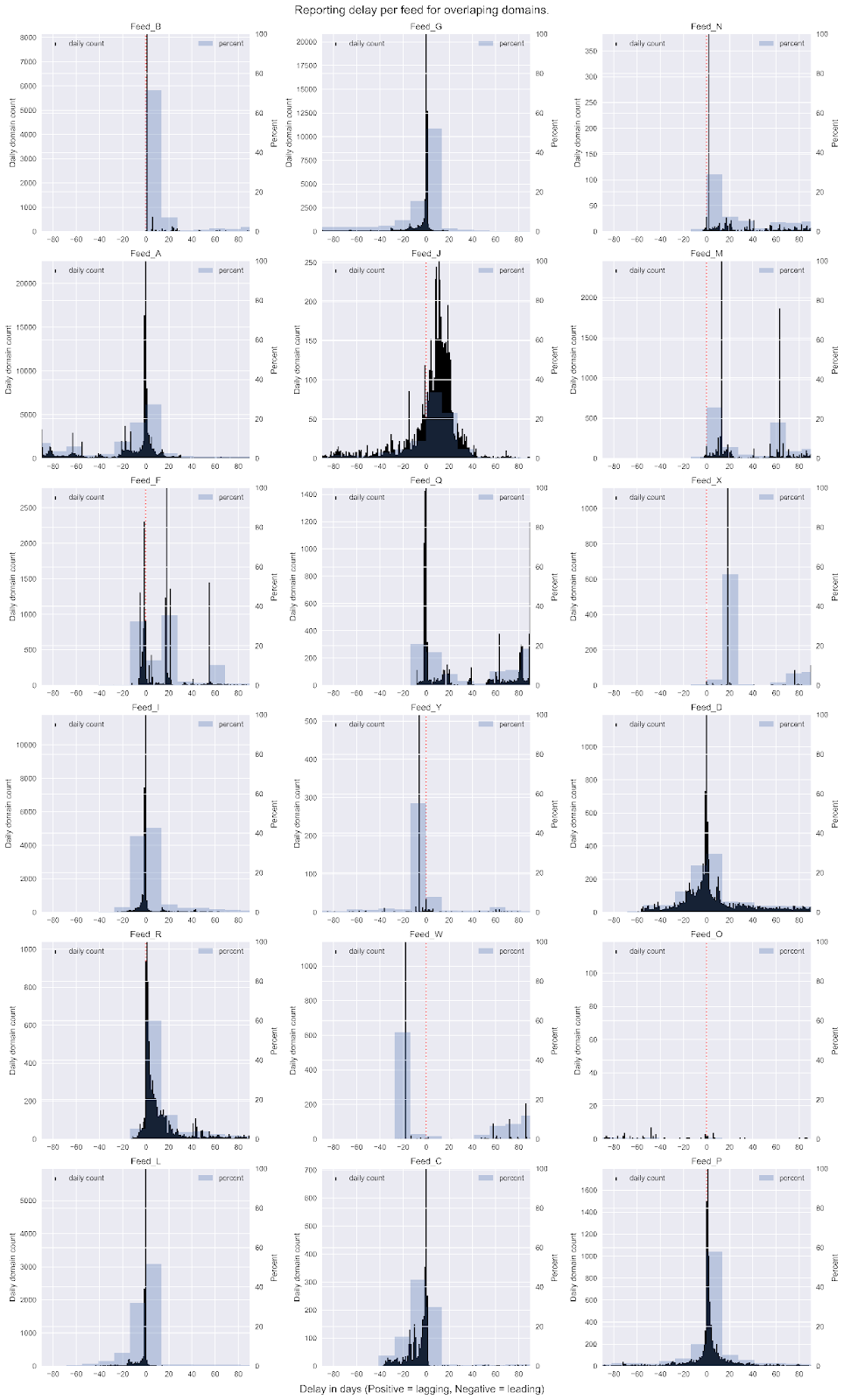
Figure 6. Delay in reporting time between each feed. Daily delay count is shown in black stems. Percentages are shown by blue bars. 0 days is shown by the red dotted line.
Utilizing automated analysis techniques
This article shows a small number of analysis techniques that can be applied to a collection of threat data. As automation is increasingly used to generate threat data, it must also be used to evaluate it.
These analyses can reveal blindspots, redundancy, and value-for-money opportunities, and even technical issues. This helps make our selection of threat feed data as beneficial to our customers as possible by:
- Increasing our coverage of the totality of active cyber threats
- Reducing redundancy among feeds
- Ensuring threat data is timely
- Ensuring cost effectiveness of the threat feeds we purchase
Of course human analysis can never be replaced particularly when searching for ground truth, but these techniques can reveal where to start looking.
Share this
Categories
- Featured (258)
- Protective DNS (15)
- IT (12)
- Cybersecurity Brief (7)
- AI (6)
- Deep Dive (6)
- IndyCar (6)
- Content Filtering (5)
- Public Wi-Fi (5)
- IT Challenges (4)
- Roaming Client (4)
- Team (4)
- Compare (3)
- Malware (3)
- Tech (3)
- Anycast (2)
- Events (2)
- Machine Learning (2)
- Phishing (2)
- Ransomware (2)
- Tech Stack (2)
- Secure Web Gateway (1)
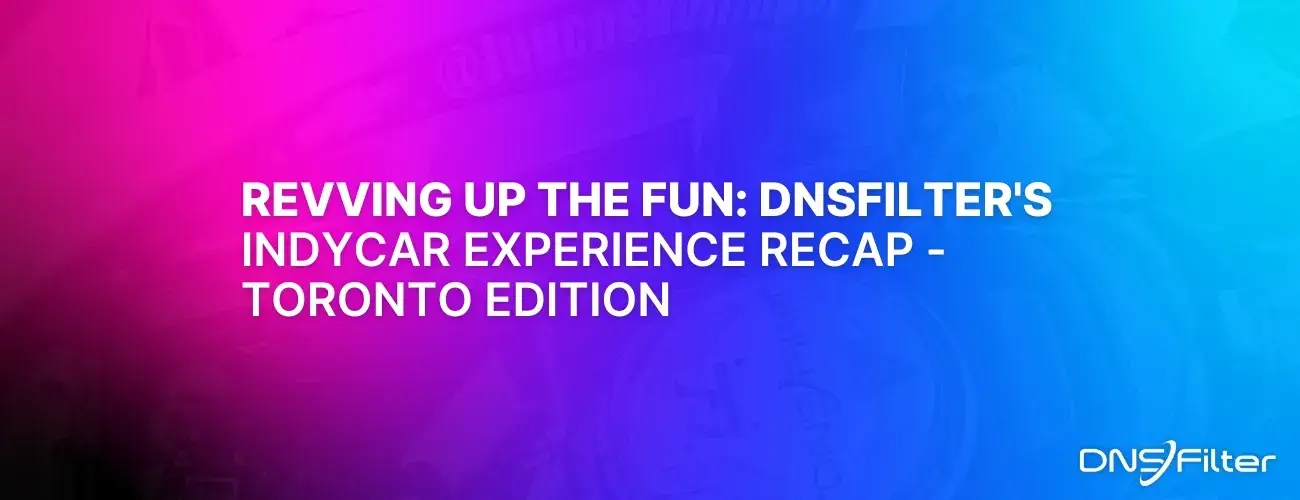
First raced in 1918, the Toronto Grand Prix is an exciting and long-standing IndyCar tradition. With 11 turns winding through downtown Toronto, including a particularly tight final curve, this street course always lends to a competitive and unpredictable race.
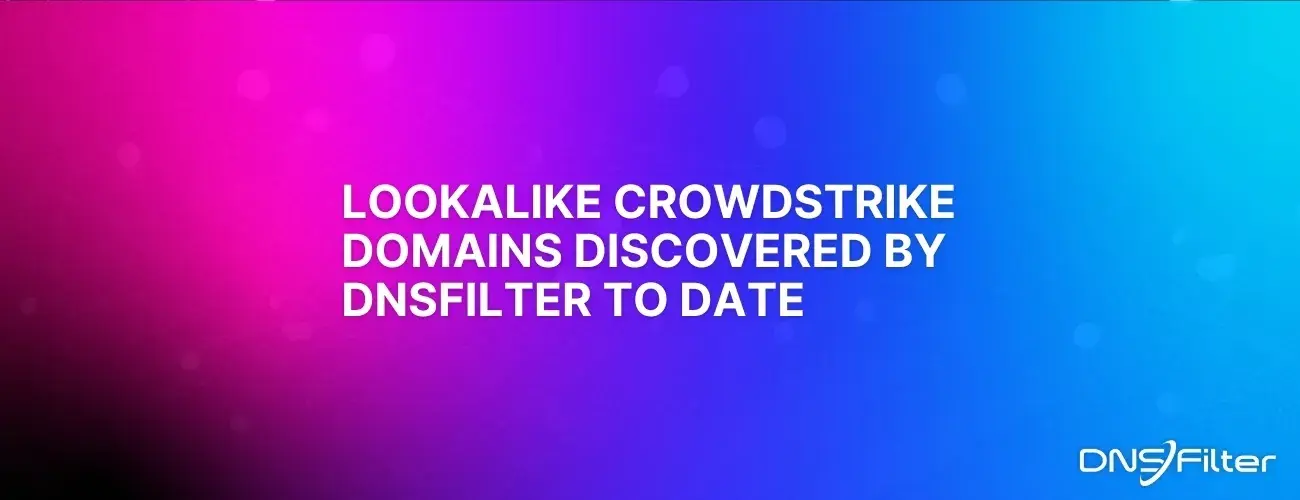
As most of the world is aware, after a routine update pushed by CrowdStrike last week to Microsoft devices, a global IT outage occurred impacting a range of industries including hospitals, broadcast television, government, and most notoriously airlines.
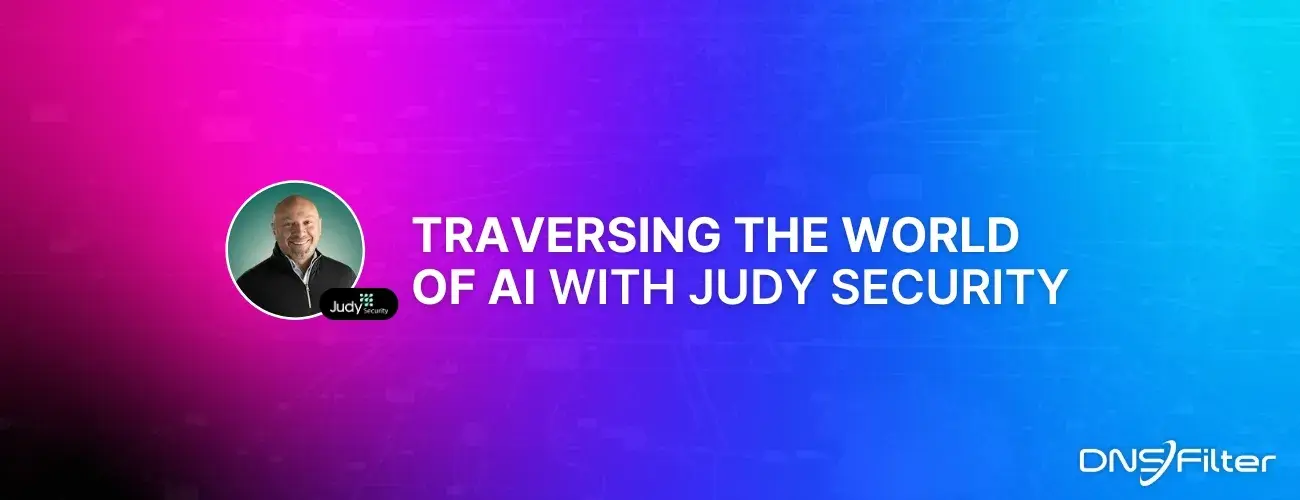
Raffaele Mautone, CEO of Judy Security, recently joined us for an interview session around the increasing presence of AI in cybersecurity. This insightful Q&A session sheds light on how AI is integrated into Judy Security's operations. Raffaele also touches on the broader implications of AI for the future, making a compelling case for its strategic use in both day-to-day operations and long-term security strategies.